AJAI vs. Machine Studying: Understanding the Key Differences
Artificial Intelligence (AI) and Machine Understanding (ML) are two of the most significant fields driving technological developments today. They are generally mentioned together, but are unique concepts that have fun different roles throughout the world involving modern technology. Even though Machine Learning is a subset of Artificial Intelligence, it’s vital to understand the essential differences between the two to understand exactly how they contribute in order to the progress brilliant systems. On this page, we’ll explore the descriptions of AI plus ML, their important differences, and exactly how they will work together in order to shape the prospect of technology.
a single. What is Synthetic Intelligence (AI)?
Man-made Intelligence (AI) appertains to the development of personal computer systems or machines that can perform tasks that usually require human intelligence. AI systems are made to mimic human cognitive functions such as learning, reasoning, problem-solving, perception, and decision-making. The aim of AI is to create equipment that can think, learn, and adjust to solve complex difficulties autonomously.
AI can be classified into two sorts:
Narrow AI (Weak AI): This variety of AI will be designed to conduct a specific task or a narrow range of duties. Such as, voice co-workers like Siri or even Alexa, recommendation systems on streaming websites like Netflix, and facial recognition software are typical examples associated with Narrow AI. These systems are quite specialized but do not necessarily possess general intelligence beyond their set functions.
General AI (Strong AI): General AI is a new theoretical concept in which machines would have typically the ability to realize, learn, and apply knowledge across some sort of wide variety involving tasks, much just like human intelligence. This kind of level of AJAI does not however exist and is still a topic of continuing research.
AI is usually not limited to merely one technology; it encompasses various career fields, such as machine learning, natural language processing (NLP), computer system vision, and robotics. AI systems may be used in a number of applications, from autonomous vehicles to health-related diagnostics and customer satisfaction.
2. What will be Machine Learning (ML)?
Machine Learning (ML) is actually a subset of Artificial Intelligence that focuses specifically upon building algorithms and even models that let computers to understand coming from data. Unlike traditional AI systems, which usually require explicit development to perform a job, ML enables techniques to improve their very own performance over moment by recognizing styles in data and making predictions or decisions based upon that data.
ML runs by training a model on large datasets, allowing it to be able to identify trends, correlations, and patterns. While the model is definitely exposed to additional data, it will become better at making predictions or getting actions. You can find a few main types of machine learning:
Supervised Learning: In supervised understanding, the model is definitely trained on a tagged dataset, the location where the insight data plus the corresponding correct outputs will be provided. The goal is for the particular model to study the relationship between your inputs and outputs so that it can make correct predictions on brand-new, unseen data. Frequent applications include distinction and regression jobs.
Unsupervised Learning: Throughout unsupervised learning, typically the model is given data without marked outputs. The goal is to find concealed patterns or types in the data. Clustering and association are common techniques employed in unsupervised mastering.
Reinforcement Learning: Encouragement learning involves teaching an agent in order to make decisions depending on feedback from their environment. The real estate agent receives rewards or penalties based in its actions in addition to learns to enhance its behavior over time. This kind of learning is definitely used in software like robotics, video gaming, and autonomous vehicles.
Machine Learning will be widely used throughout industries like finance, healthcare, e-commerce, plus entertainment for responsibilities for instance fraud detection, recommendation systems, graphic recognition, and predictive analytics.
3. Essential Differences Between AI and Machine Learning
Although AI plus ML are closely related, they possess distinct characteristics that differentiate them through each other. Let’s explore the essential differences:
1. Range and Definition
AI: Artificial Intelligence is definitely the broader idea of creating smart machines that may perform tasks that will would typically require human intelligence. AJE encompasses a broad variety of technologies, including logic, problem-solving, reasoning, and arranging. It is certainly not restricted to learning by data and also includes cognitive processes such as understanding, perception, plus decision-making.
ML: Machine Learning is actually a subset of AI targeted on the ability of machines in order to learn from data and enhance their performance over time. ML involves creating algorithms that allow methods to recognize patterns and make decisions based on past encounters (data), instead of based on explicit programming.
2. Learning Procedure
AI: Traditional AJAI systems are designed to follow predefined rules or codes to perform tasks. These systems do not "learn" in typically the sense that ML systems do. Rather, they execute duties based on set instructions created by humans.
ML: Machine Learning, on typically the other hand, entails listening to advice from data. MILLILITERS algorithms improve their own performance because they are exposed to more data and experience, establishing over time to be able to make better intutions or decisions.
three or more. Dependence on Files
AI: AI devices can work together with or without files, depending on the particular task. For example, rule-based AI systems can operate using human-defined logic or selection trees without the need for large datasets. These types of systems work inside situations where thought and logic enjoy a central function.
ML: Machine Studying, by definition, demands data. ML algorithms rely heavily upon large datasets to get patterns, make predictions, and continuously enhance. The quality and amount of data usually are critical for training correct ML models.
four. Decision-Making
AI: AJE systems may make use of a combination associated with reasoning, decision trees and shrubs, and predefined regulations to arrive at decisions. These types of decisions are generally deterministic, and therefore typically the same input will usually lead to the particular same output.
CUBIC CENTIMETERS: Machine Learning models make decisions structured on statistical evaluation of data. Typically the decisions are probabilistic, meaning that the one could make slightly different predictions everytime, even with the exact same input, based in the patterns it includes learned from the particular data.
5. Intricacy and Application
AJE: AI systems usually are typically more advanced because they aim in order to replicate human intellect. AI can be used for a comprehensive portfolio of tasks that entail reasoning, perception, problem-solving, and planning. https://outsourcetovietnam.org/data-science-vs-artificial-intelligence-vs-machine-learning/ For example self-driving cars, smart virtual assistants, plus medical diagnostic methods.
ML: Machine Mastering is focused upon specific tasks these kinds of as prediction, distinction, and clustering. That is particularly helpful for problems where a lot of data happen to be available and designs need to be uncovered. Common programs of ML consist of recommendation engines, picture recognition, and speech-to-text systems.
4. Exactly how AI and Machine Learning Interact
Although AI and Machine Learning are unique fields, they frequently function together to create intelligent systems. Inside many modern AJAI applications, machine learning plays a key role in enabling systems to understand by data create selections. For example:
AJAI in Healthcare: AI systems use ML models to examine medical images plus predict patient outcomes according to large datasets of medical records. The ML methods process the info to identify designs, while AI devices help interpret plus act on the results, making selections that assist medical doctors in diagnosing illnesses.
AI in Independent Vehicles: Self-driving cars use AI to be able to process real-time data from cameras, receptors, and maps. Machines Learning algorithms will be used to acknowledge objects, make predictions about traffic styles, and optimize generating behavior, allowing the particular car to get around safely and autonomously.
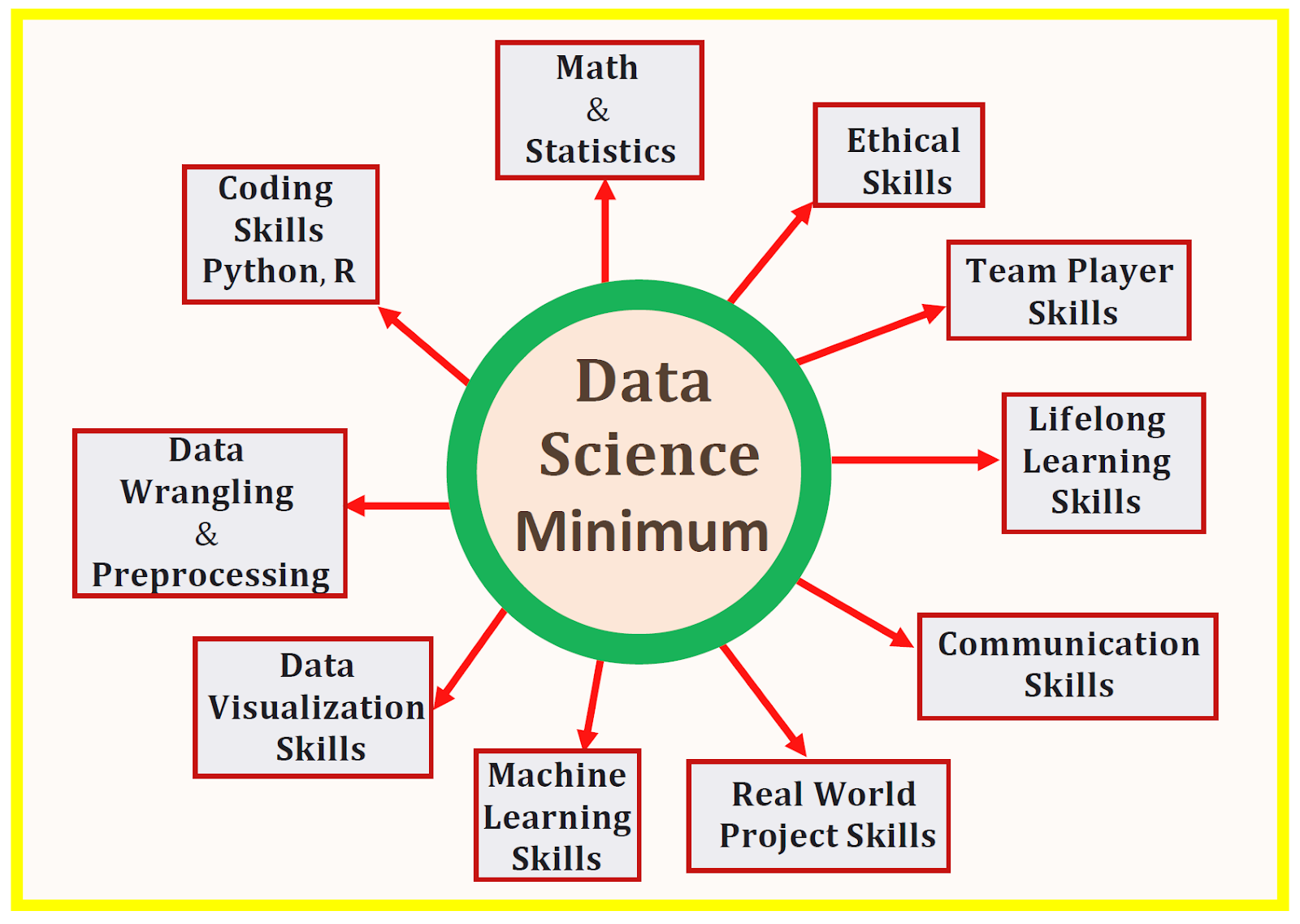
AI in Customer satisfaction: AI-powered chatbots work with machine learning codes to understand and even respond to customer requests. The equipment learning top models improve over time period by analyzing previous interactions and mastering how to act in response more effectively.
5. The Future of AJE and Machine Studying
As AI plus ML technologies carry on and evolve, their programs will expand directly into new areas, ultimately causing more sophisticated techniques that can perform more and more complex tasks. AI and ML will carry on and drive advancement in industries these kinds of as healthcare, finance, manufacturing, and enjoyment.
Some key tendencies to watch intended for include:
Explainable AJE (XAI): As AJE systems become more complex, there is a growing need for transparency in just how decisions are produced. Explainable AI aspires to make AJE models more interpretable, helping humans understand how the system found a certain decision.
AI plus ML in Edge Computing: Using the rise of Internet involving Things (IoT) gadgets, AI and ML will increasingly be used at the edge, where data is definitely processed locally on devices rather as compared to sent to the particular cloud. This may enable faster decision-making and real-time answers in areas such as autonomous driving plus smart homes.
Values and Fairness: As AI and CUBIC CENTIMETERS are used in more critical applications, this sort of as hiring selections, law enforcement, plus healthcare, ensuring that these systems happen to be ethical, fair, and even unbiased is a major focus within the coming years.
Summary
Found in summary, while Unnatural Intelligence (AI) and even Machine Learning (ML) are related, they are not the same. AI is a broader strategy that encompasses numerous technologies aimed with replicating human intellect, whereas Machine Mastering is a subset of AI that focuses on running machines to understand from data. CUBIC CENTIMETERS is an fundamental tool for developing intelligent systems that could improve over time period by recognizing styles in data, although AI also includes other strategies of decision-making and problem-solving that go beyond listening to advice from data. By learning the key differences among AI and ML, we can far better appreciate how these kinds of technologies complement each and every other to create powerful, intelligent systems that are transforming sectors worldwide.